Bring this project to life
In the era of Gen-AI, you now have the opportunity to experiment with various hairstyles and craft a new look for yourself. Whether you are considering a dramatic change or just wanting to refresh your style, the process of envisioning yourself with a different hairstyle can be both thrilling and intimidating. However, with the aid of artificial intelligence (AI) technology, the realm of hairstyling transformations is undergoing a revolutionary evolution.
Imagine having the ability to explore a limitless range of hairstyles, from timeless cuts to nostalgic 90’s designs, all from the convenience of your own home. This futuristic dream is now a tangible reality thanks to AI-powered virtual hairstyling platforms. By harnessing the power of advanced algorithms and machine learning, these innovative platforms enable users to virtually try on various hairstyles in real-time, offering a seamless and immersive experience unlike any other.
In this post, we will delve into HairFastGAN and delve into how AI is reshaping our approach to experimenting with hairstyles. Whether you are a beauty enthusiast eager to explore new trends or someone considering a bold hair transformation, join us on a journey through the exciting realm of AI-driven virtual hairstyles.
Introduction
This article introduces HairFast, a groundbreaking model designed to simplify the intricate task of transferring hairstyles from reference images to personal photos for virtual try-on. Unlike existing methods that are either slow or compromise on quality, HairFast excels in speed and reconstruction precision. By operating in StyleGAN’s FS latent space and incorporating enhanced encoders and inpainting techniques, HairFast achieves high-resolution results in near real-time, even when faced with challenging pose differences between source and target images. This approach surpasses existing methods, delivering remarkable realism and quality, even when transferring hairstyle shape and color in less than a second.
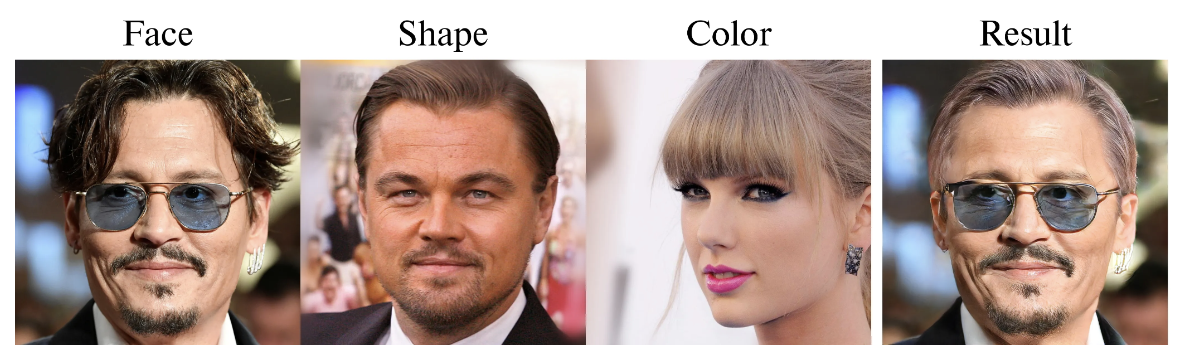
Thanks to advancements in Generative Adversarial Networks (GANs), we can now utilize them for semantic face editing, including hairstyle changes. Hairstyle transfer is a particularly complex and intriguing aspect of this field. It involves extracting features like hair color, shape, and texture from one image and applying them to another while preserving the individual’s identity and background. Understanding how these attributes interact is crucial for achieving desirable outcomes. This type of editing has numerous practical applications, whether you are a professional working with photo editing software or an individual engaging in virtual reality or computer games.
The HairFast method offers a swift and high-quality solution for altering hairstyles in images. It can handle high-resolution images and produces results comparable to the best existing methods. Moreover, it is efficient enough for interactive usage, thanks to its adept use of encoders. This method operates in four steps: embedding, alignment, blending, and post-processing. Each step is handled by a specialized encoder trained for its specific task.
Recent advancements in Generative Adversarial Networks (GANs), such as ProgressiveGAN, StyleGAN, and StyleGAN2, have significantly enhanced image generation, especially in creating highly realistic human faces. However, achieving high-quality, fully controlled hair editing remains a challenge due to various complexities.
Different methods approach this challenge differently. Some focus on balancing editability and reconstruction fidelity through latent space embedding techniques, while others, like Barbershop, break down the hair transfer task into embedding, alignment, and blending subtasks.
Approaches like StyleYourHair and StyleGANSalon aim for greater realism by integrating local style matching and pose alignment losses. Meanwhile, HairNet and HairCLIPv2 tackle complex poses and diverse input formats.
Encoder-based methods, such as MichiGAN and HairFIT, accelerate runtime by training neural networks instead of using optimization processes. CtrlHair, a standout model, uses encoders to transfer color and texture, but still grapples with complex facial poses, resulting in slow performance due to inefficient post-processing.
Overall, despite significant progress in hair editing using GANs, challenges remain to achieve seamless and efficient results in various scenarios.
Methodology Overview
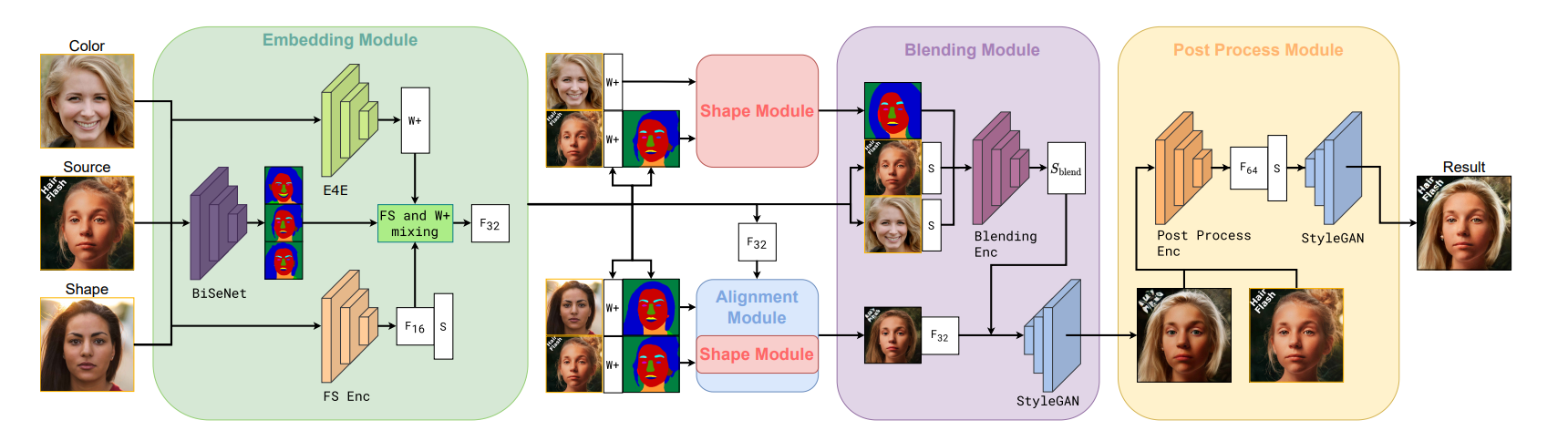
This innovative method for transferring hairstyles mirrors the Barbershop approach; however, all optimization processes are replaced with trained encoders for enhanced efficiency. In the Embedding module, representations of original images are captured in StyleGAN spaces, such as W+ for editing and FS space for detailed reconstruction. Additionally, face segmentation masks are utilized for later stages.
Transitioning to the Alignment module, the shape of the hairstyle from one image to another is primarily achieved by focusing on altering the tensor F. Here, two tasks are accomplished: generating the desired hairstyle shape through the Shape Module and adjusting the F tensor for inpainting post-shape alteration.
In the Blending module, the transition of hair color from one image to another is executed. By modifying the S space of the source image using the trained encoder, this is accomplished while considering additional embeddings from the source images.
Although the image post-blending could be deemed final, a new Post-Processing module becomes necessary. This step aims to recuperate any lost details from the original image, ensuring facial identity preservation and method realism enhancement.
Embedding
To initiate a hairstyle transformation, images are first converted into StyleGAN space. Methods like Barbershop and StyleYourHair achieve this by reconstructing each image in FS space through an optimization process. However, in this study, a pre-trained FS encoder is employed to swiftly provide the FS representations of images. It is considered one of the top encoders available and yields visually appealing images.
However, there is a challenge: FS space poses difficulties for hair color alterations when using the FS encoder in Barbershop. To address this issue, another encoder called E4E is utilized. Although it is simpler and not as proficient at enhancing image aesthetics, it excels at facilitating alterations. Subsequently, the F tensor (which contains hair-related information) from both encoders is blended to resolve this issue.
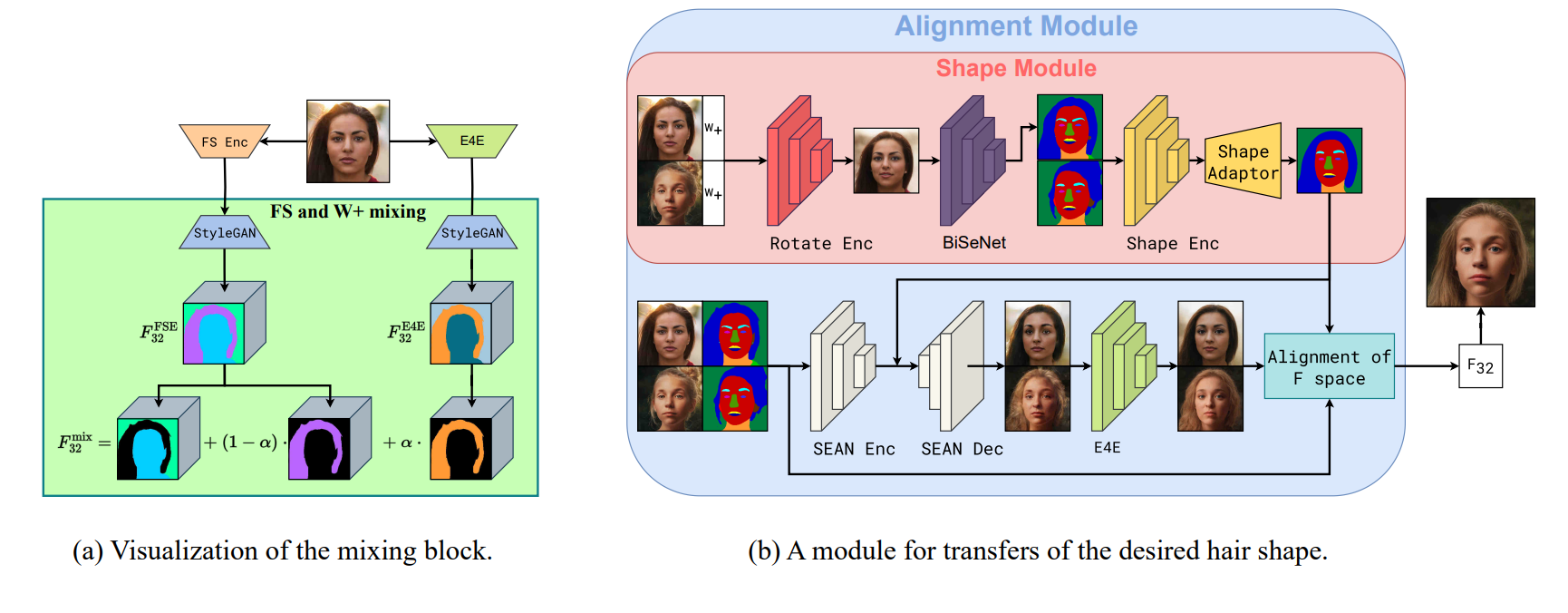
Alignment
During this phase, the hair transformation occurs, ensuring that the hair in one image matches the hair in another image. A mask outlining the hair is created, and the hair in the first image is adjusted to align with that mask.
A method known as CtrlHair has been devised to accomplish this task. It utilizes a Shape Encoder to comprehend the shapes of hair and faces in images, along with a Shape Adaptor to align the hair in one image to match the shape of another. While this method generally performs well, it encounters challenges.
One significant challenge is that the Shape Adaptor is trained to manage hair and faces in similar poses. Therefore, if the poses differ significantly between the two images, it can distort the hair’s appearance. The CtrlHair team attempted to resolve this issue by adjusting the mask post-alignment, but this solution is not the most efficient. To address this issue, a new tool called Rotate Encoder has been developed. It is trained to adjust the shape image to align with the source image’s pose. This is primarily achieved by modifying the image’s representation before segmentation. There is no need for fine-tuning the details for mask creation, hence a simplified representation is employed in this scenario. This encoder is adept at handling complex poses without distorting the hair. If the poses already match, it will not disrupt the hairstyles.
Blending
In the subsequent step, the primary focus is on altering the hair color to the desired hue. Previously, Barbershop’s approach was rigid, attempting to strike a balance between the source and desired color vectors. This often led to incomplete edits and introduced unwanted artifacts due to outdated optimization techniques.
To enhance this process, a similar encoder architecture named HairCLIP is introduced, predicting how the hair vector style changes when provided with two input vectors. This method employs special modulation layers that are more stable and effective for style alterations.
Additionally, our model receives CLIP embeddings of both the source image (including hair) and the hair-only section of the color image. This additional information aids in preserving details that may be lost during the embedding process and has been proven to significantly enhance the final outcome based on our experiments.
Experiments Results
The experiments demonstrated that while the CtrlHair method scored highest based on the FID metric, it did not visually outperform other state-of-the-art approaches. This disparity arises from its post-processing technique, which involved blending the original image with the final result using Poisson blending. While this method was favored by the FID metric, it often resulted in noticeable blending artifacts. On the contrary, the HairFast method exhibited a superior blending process but encountered difficulties when significant changes in facial hues were present. This made utilizing Poisson blending effectively challenging, as it tended to accentuate shade disparities, resulting in lower quality metric scores.
A novel post-processing module has been developed in this study, serving as an advanced tool for image enhancement. It is designed to handle intricate tasks, such as reconstructing the original face and background, refining hair after blending, and filling in any missing elements. This module generates highly detailed images, with four times more detail than previous methods used. Unlike other tools focused on image editing, ours prioritizes achieving the best possible image quality without necessitating further alterations.
Demo
Bring this project to life
To run this demo, first open the notebook HairFastGAN.ipynb
. This notebook contains all the necessary code to experiment with the model. To execute the demo, you need to clone the repository and install the required libraries accordingly.
- Clone the repository and install Ninja
!wget https://github.com/ninja-build/ninja/releases/download/v1.8.2/ninja-linux.zip
!sudo unzip ninja-linux.zip -d /usr/local/bin/
!sudo update-alternatives --install /usr/bin/ninja ninja /usr/local/bin/ninja 1 --force
## clone repo
!git clone https://github.com/AIRI-Institute/HairFastGAN
%cd HairFastGAN
- Install necessary packages and pre-trained models
from concurrent.futures import ProcessPoolExecutor
def install_packages():
!pip install pillow==10.0.0 face_alignment dill==0.2.7.1 addict fpie \\
git+https://github.com/openai/CLIP.git -q
def download_models():
!git clone https://huggingface.co/AIRI-Institute/HairFastGAN
!cd HairFastGAN && git lfs pull && cd ..
!mv HairFastGAN/pretrained_models pretrained_models
!mv HairFastGAN/input input
!rm -rf HairFastGAN
with ProcessPoolExecutor() as executor:
executor.submit(install_packages)
executor.submit(download_models)
- Set up an argument parser and use the script for downloading, converting, and displaying images
import argparse
from pathlib import Path
from hair_swap import HairFast, get_parser
model_args = get_parser()
hair_fast = HairFast(model_args.parse_args([])
- Try the hair swap with the downloaded image
input_dir = Path('/HairFastGAN/input')
face_path = input_dir / '6.png'
shape_path = input_dir / '7.png'
color_path = input_dir / '8.png'
final_image = hair_fast.swap(face_path, shape_path, color_path)
T.functional.to_pil_image(final_image).resize((512, 512)) # 1024 -> 512
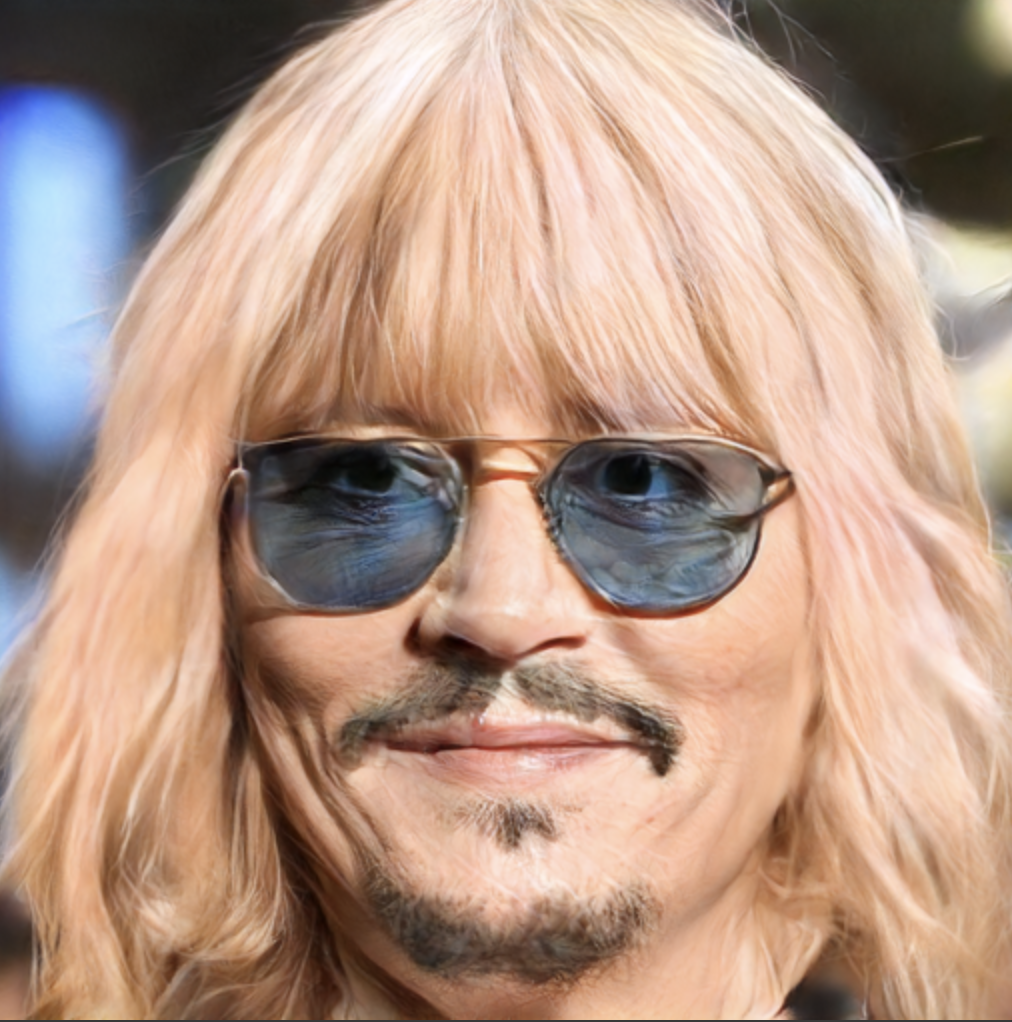
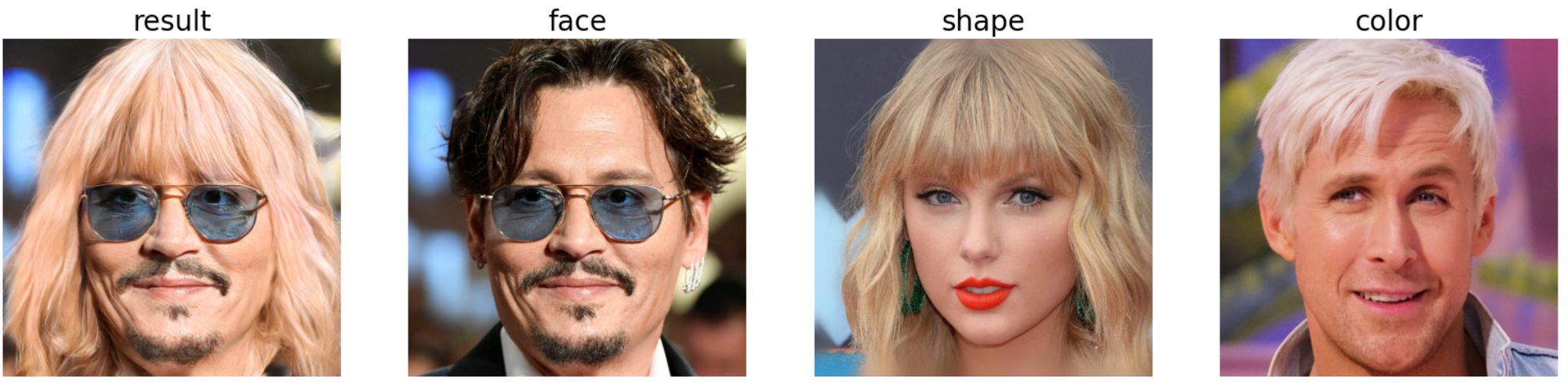
Ending Thoughts
In this article, we introduced the HairFast method for hair transfer, notable for delivering high-quality, high-resolution outcomes akin to optimization-based techniques while operating at nearly real-time speeds.
Despite the limitations in the ways hairstyles can be transferred, this method paves the way for addressing this constraint in future endeavors.
Moreover, the future of virtual hair styling using AI holds tremendous potential for reshaping how we engage with and explore hairstyles. With advancements in AI technologies, even more realistic and personalized virtual hair makeover tools are on the horizon. Consequently, this will lead to highly tailored virtual styling experiences