Artificial Intelligence (AI) is a combination of various programs and technologies, including Machine Learning and large language models. These components enable AI to perform tasks independently without human intervention.
The increasing interest and advancement in AI agents and traditional AI technologies highlight their crucial roles in enhancing work efficiency, resource management, and productivity across different industries.
Although both AI agents and traditional AI leverage human-like intelligence, they differ significantly in features and use cases. This article offers a detailed comparative analysis of AI agents vs. Traditional AI, focusing on their approaches to autonomy and decision-making.
Overview of Traditional AI
Traditional AI, also known as rule-based or standard AI, is an earlier generation of AI systems that rely on pre-set algorithms and a defined set of rules to execute specific tasks. These systems operate in structured environments where users provide explicit instructions, limiting their adaptability and problem-solving abilities.
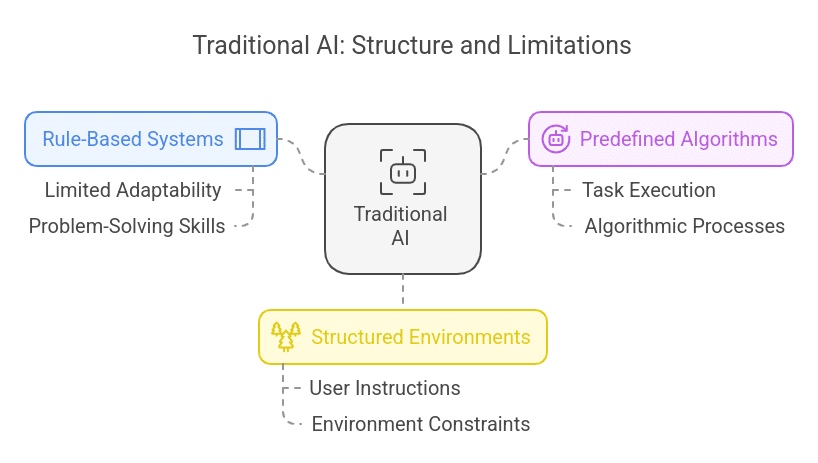
For example, traditional chatbots follow specific inputs and offer predefined options. If users choose from these options, the chatbots proceed to the next task; otherwise, they repeat the available options. However, these traditional AI systems do not learn or improve over time and can only operate within their predefined framework.
Introduction to AI Agents
AI agents represent the latest generation or upgraded version of traditional AI. They collect data by perceiving their environment and autonomously execute appropriate actions by learning and acquiring information to achieve their goals.
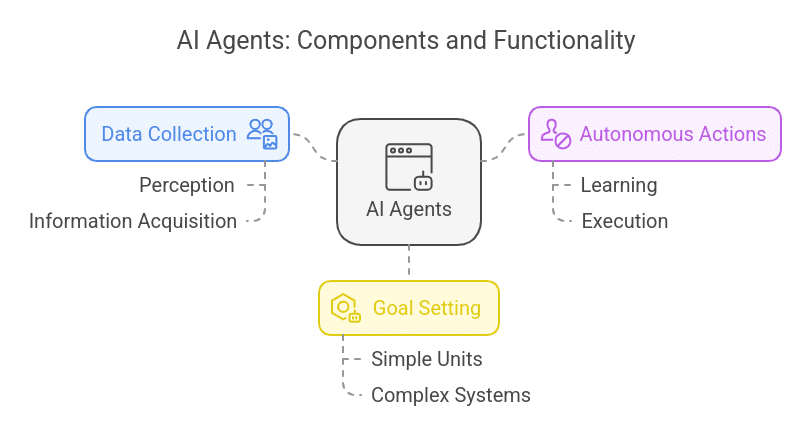
AI agents are autonomous software programs that continuously enhance their performance over time. Users only need to define a goal, and the AI agents determine the best course of action to achieve it. These agents can range from simple units to complex systems capable of performing multiple actions simultaneously.
Popular examples of AI agents include AI assistants like Alexa, Gemini, and Siri. These agents interact with users, learn new information or skills, and provide immersive user experiences. They retrieve relevant information from their database to respond to user queries and can learn new skills based on user interactions.
In addition to AI assistants, AI agents are utilized in technologies such as big data analytics, machine learning model development, and predictive analytics.
AI Agents vs. Traditional AI: Comparative Analysis
Several factors can be used to compare AI agents and Traditional AI, including autonomy, learning capabilities, and adaptability. The following sections outline the key differences between the two based on these factors.
Autonomy
Autonomy in AI Agents
AI agents possess complex algorithms that enable them to operate autonomously and make decisions without constant user instructions. They can learn from previous experiences and adapt their actions based on environmental stimuli, enhancing their decision-making skills over time.
Autonomy in Traditional AI
In contrast, traditional AI systems often require human intervention for decision-making and operations. They rely on predefined rules and instructions to perform tasks, lacking the autonomy and adaptability of AI agents.
Learning Capabilities
Learning Capabilities of AI Agents
AI agents can continuously learn and adapt to new strategies based on progressive machine learning algorithms. They excel in dynamic environments where adaptation is crucial.
Learning Capabilities of Traditional AI
Traditional AI systems have limited learning capabilities as they follow fixed sets of rules and commands. They require human intervention to update instructions and improve performance.
Adaptability
Adaptability in AI Agents
AI agents exhibit high adaptability by interacting with their environment, learning from experiences, and adjusting their actions to dynamic changes. They can effectively adapt to new strategies based on current data.
Adaptability in Traditional AI
Traditional AI systems have limited adaptability as they rely on fixed rules and instructions without changing behavior based on responses. They provide consistent performance within their framework but struggle to adapt to new scenarios.
AI Agents vs. Traditional AI: Use Cases
Use Cases for Traditional AI
Traditional AI finds applications in various industries such as credit score checking, spam email filters, healthcare devices, e-commerce platforms, and logistics and supply chains. These systems excel in tasks requiring precision and reliability.
Use Cases for AI Agents
AI agents are versatile in industries like web design, finance, healthcare, e-commerce, and automobile sectors. They enhance customer experiences, optimize processes, improve security, and provide real-time insights for decision-making.
Conclusion
AI agents and traditional AI exhibit distinct functionalities, with AI agents offering greater autonomy, adaptability, and continuous learning capabilities. While traditional AI is cost-effective and precise for specific tasks, AI agents provide flexibility and efficiency, making them more suitable for dynamic environments. The role of AI agents in enhancing human life will continue to evolve and expand.