Introduction
Artificial intelligence (AI) is transforming our work, lives, and interaction with technology. Two critical subfields of AI, Generative AI, and Predictive AI, are driving innovation in the field. While both rely on data and complex algorithms, their core functions differ significantly.
Predictive AI focuses on forecasting future events, while Generative AI is designed to generate new data or content based on learned patterns. In this article, we will delve into the functionalities, distinctions, and real-world applications of both Generative AI and Predictive AI.
What is Generative AI?
Generative AI is a branch of artificial intelligence that creates new content—whether it be text, images, audio, or code—by learning patterns from existing data. By mimicking the patterns and characteristics of the data they are trained on, these systems produce outputs that are authentic and natural.
Explore more about what Generative AI entails.
What is Predictive AI?
Predictive AI is a facet of artificial intelligence that focuses on predicting future events or outcomes based on historical or real-time data. It employs algorithms such as regression, classification, and time-series analysis to identify patterns and make informed predictions about future occurrences.
The primary goal of Predictive AI is to forecast future trends or behaviors by analyzing past data and identifying patterns. Its main objective is to provide reliable predictions that can guide decision-making across various domains.
How does Generative AI Work?
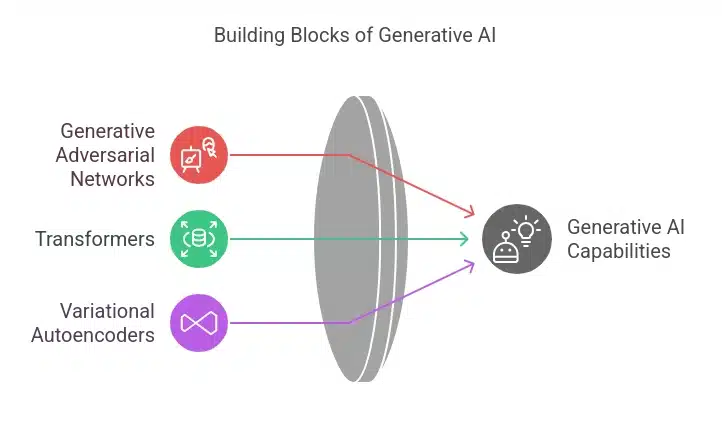
Generative AI utilizes sophisticated machine learning techniques such as:
- Generative Adversarial Networks (GANs): GANs consist of a discriminator and a generator. The discriminator evaluates the generator’s output against real data, enhancing the quality of generated output.
- Transformers: Transformers are pivotal for natural language processing, including models like GPT. They are instrumental in creating language models that mimic human writing.
- Variational Autoencoders: VAEs compress and reconstruct data, enabling models to learn essential data features.
Learn more about Machine Learning here.
How Does Predictive AI Work?
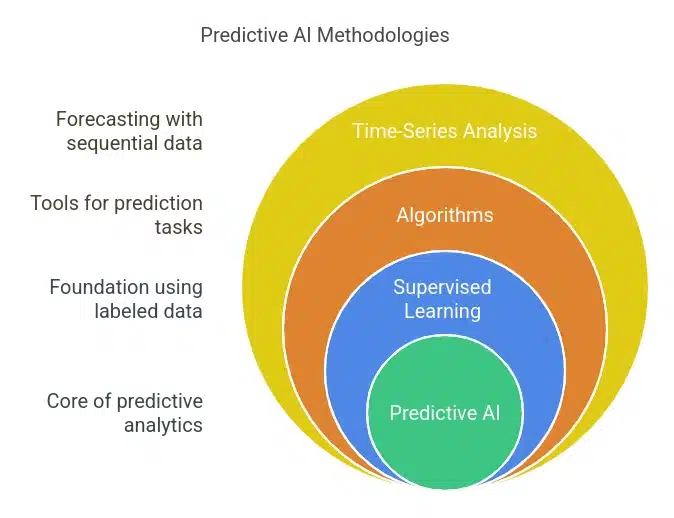
Predictive AI relies on:
- Supervised Learning: Training models on labeled datasets with known outcomes.
- Regression and Classification: Utilizing algorithms like neural networks and decision trees for prediction tasks.
- Time-Series Analysis: Analyzing sequential data to forecast future values such as sales or stock prices.
Generative AI Applications
- Content Creation:
- Tools like ChatGPT generate various types of content including blog articles, essays, and social media posts, aiding content teams in scaling their output.
Explore Generative AI Models here.
Predictive AI Applications
- Customer Churn Analysis:
- Predictive models identify customers likely to churn, enabling businesses to implement targeted retention strategies.
Difference Between Generative AI and Predictive AI
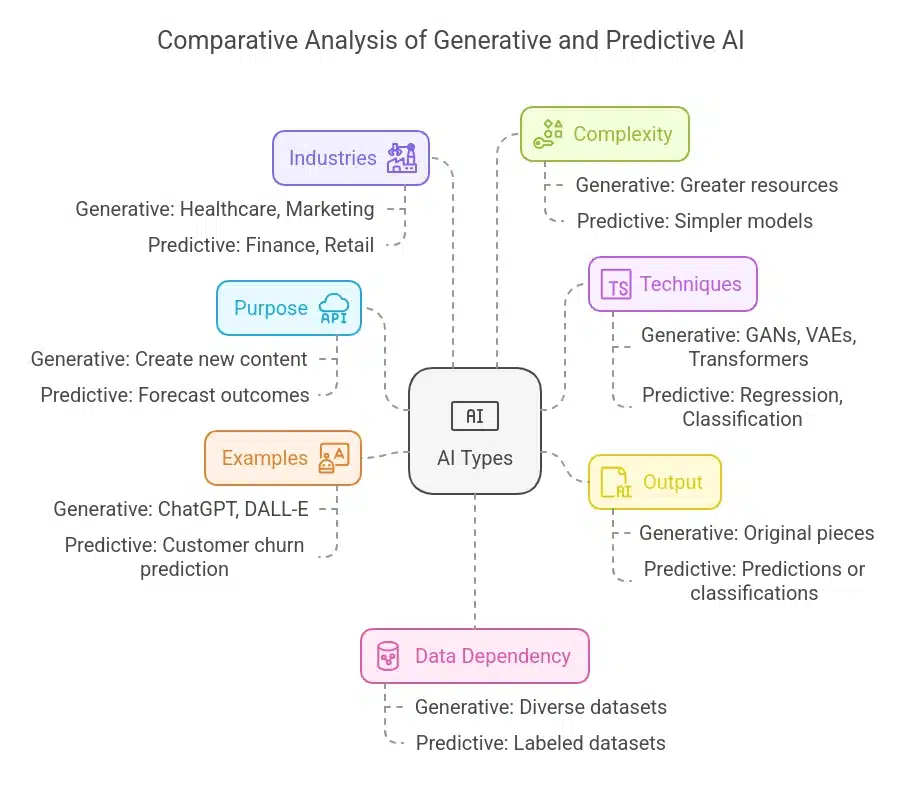
How Generative and Predictive AI Work Together?
In certain scenarios, Generative and Predictive AI complement each other:
Ethical Considerations
Challenges with Generative AI
Challenges with Predictive AI
Conclusion
Generative and Predictive AI are integral components of artificial intelligence, each serving distinct purposes. While Predictive AI excels at making accurate predictions based on historical data, Generative AI focuses on creating fresh and innovative content.
To delve deeper into AI technologies through practical projects, consider enrolling in the PG Program in AI & Machine Learning by Great Learning in partnership with UT Austin. Additionally, explore our free AI courses for foundational knowledge.
Quiz Time